Ashley Collimore, Ph.D.
Postdoctoral Associate
Multimodal, Task Aware Movement Quality Assessment and Activity Recognition
Project Background
​
Current assistive technologies for individuals post-stroke are passive and only provide assistance on flat, level ground or are active but require user input to switch between assistive modes. The long term goal of this work is to develop a minimal-sensor system that can detect a user's activities and automatically switch between modes on a powered device, such as a soft-robotic exosuit or a functional electrical stimulation system.
As an intermediary step, we propose developing a system that can be used to a) automatically score movement assessments and b) be deployed in the home to track changes in movement function over time.
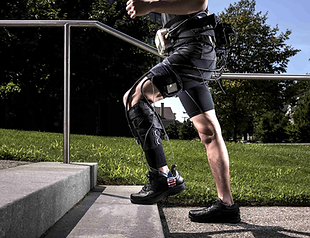

Preliminary Work
​
We collected data from 5 neurologically-intact individuals using inertial measurement units, a depth camera, and a motion capture system. We developed an algorithm to combine IMU and depth camera data and compared the human tracking results to the motion capture, demonstrating that our algorithm was more accurate than the current state-of-the-art. This work was recently accepted to the 2021 IEEE International Conference of Robotics and Automation and will be presented in May 2021.
Publications
Mitjans M, Theofanidis M, Collimore AN, Disney ML, Levine DM, Awad LN, Tron R. Visual-Inertial Filtering for Human
Walking Quantification. IEEE International Conference on Robotics and Automation (ICRA). 2021. View the paper.
1. P. Wang, W. Li, P. Ogunbona, J. Wan, and S. Escalera, “Rgb-d-based human motion recognition with deep learning: A survey,”Computer Vision and Image Understanding, vol. 171, pp. 118–139, 2018
2. Z. Cao, G. Hidalgo, T. Simon, S.-E. Wei, and Y. Sheikh, “Openpose:realtime multi-person 2d pose estimation using part affinity fields,”arXiv preprint arXiv:1812.08008, 2018